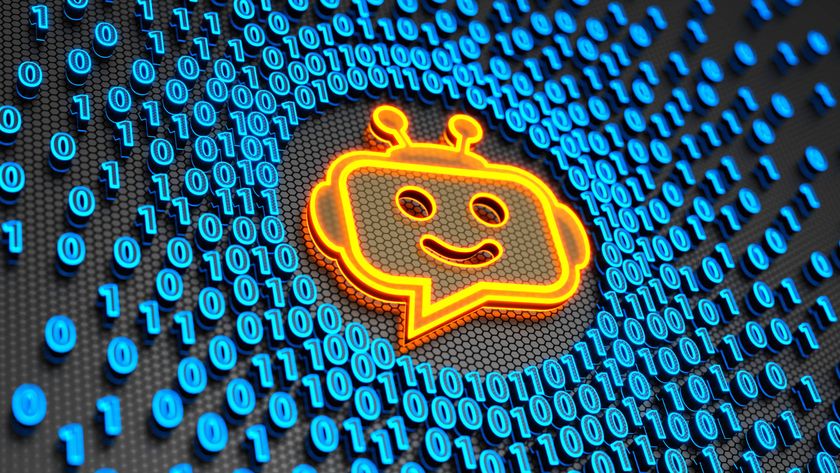
In a breakthrough in AI understanding, Anthropic has developed a novel technique known as circuit tracing, which allows researchers to monitor the decision-making processes within large language models (LLMs). This method reveals why chatbots frequently struggle with simple arithmetic and can produce misleading outputs.
The findings emphasize that while LLMs are adept at generating coherent text, their processes are often opaque. Joshua Batson, research scientist at Anthropic, explained, “Open up a large language model and all you will see is billions of numbers—the parameters. It’s not illuminating.”
This innovative approach mimics brain-scanning techniques, providing insights into parts of the model that are active during various tasks. For instance, when asked to solve math problems, LLMs take unexpected paths—an example being how Claude approximated the sum of 36 and 59 before arriving at 95.
Batson remarked, “The planning thing in poems blew me away. Instead of at the very last minute trying to make the rhyme make sense, it knows where it’s going.”
Moreover, the research signifies that LLMs don’t merely predict the next word but also employ a broader conceptual framework that may span multiple languages. Further exploration in this area is necessary as the current analysis remains labor-intensive and doesn’t elucidate how these networks operate at a foundational level.